“We must do something about
generative AI right now.”
With Copilot and GPT hitting the
market, by now most boardrooms have heard some variation of this declaration.
But while it is important for businesses to adapt quickly to new opportunities,
it is worth noting that chasing down the latest trend may sometimes end up in
wasted resources, misaligned priorities, and might even slow down the pace of
innovation. Even as business leaders give the greenlight, they must ask
themselves: As an organization, are we truly ready to adopt this trend?
A robust data analytics
foundation is a crucial precursor to Artificial Intelligence (AI) adoption. Yet
very few
businesses can confidently state that their data is ready for AI. Your
organizational data is best suited for AI when it’s cleansed, consistent, and
centrally stored. But how does one make that happen when most organizations
continue to maintain a network of disparate systems that are poorly integrated?
When data is the differentiator for success, how does one work around data
accessibility issues? When crucial information is trapped in the form of unstructured
data in presentations, strategy papers, and customer logs that are better
suited for record-keeping, not analysis, how does one fully leverage the power of
AI?
AI adoption: Go all in or take an iterative approach?
However, it is important to understand that starting the AI journey by going all out and investing in a data platform may not be the right approach for all businesses. For example, companies can take a use-case based approach to AI adoption which focuses on solving the business problem at hand, instead of focusing solely on the technology platform. A use-case driven approach can be especially beneficial as it helps businesses realize the impact and value of their investments faster. It may even be easier to drive organizational support for AI initiatives when it is a single AI use case, as opposed to when it is a complete overhaul to the technology architecture and way the organization works. Additionally, this approach allows for businesses to take a staged approach to data analytics while having a clear platform strategy identified from the beginning itself.
In a two-part blog series, we will explore how businesses can choose the right approach that works for them. This first blog will dive into what constitutes the ‘all-in’ approach, and explore how business leaders can better prepare their data platform for AI adoption to mitigate the risk of having to shelve AI projects midway. The second blog will look at how an iterative approach to AI may benefit some businesses.
Step 1: Inventorying your data
Over two-thirds of IT leaders expect data volumes to increase by 22% on average over the next year. This growing swamp of data can become a cause for concern as the rising volume and diversity of data sources means that more effort is required to standardize the data. Businesses will have to consider several key questions:
- What are the data sources we have to work with? Sources could range from databases, cloud storage, external data sources and APIs to unstructured data sources like emails, documents and sensor data.
- Where is your data located? Is it on the Cloud, on-premise, or in a combination of both?
- What type of data do you typically work with? Whether its sensitive customer information, transactional data or feeds from your sensors, your data needs to be accurately catalogued and classified based on the requirements of your business, the sensitivity of the data and your business’ unique priorities.
- How does your data fare in terms of quality? Your AI algorithms are only as good as the data it is trained on. It is important to evaluate the quality of the data in terms of accuracy, completeness, consistency, timeliness and reliability to determine its usability.
- What dependencies exist between your data sources, how often are they updated, and how does data flow from system to system?
- Are there proper permissions in place to access and read your data sources? To understand dependencies and bottlenecks, businesses must document how data is accessed, who has access to it, and for what purpose.
Step 2: Prepping your data for analysis
Anomalies, missing values and inconsistencies in your data sets can significantly skew your analytics and AI outcomes. To ensure that your data points are reliable and do not end up introducing errors into your Machine Learning (ML) algorithms, data prep is an important step. For example, missing values related to inventory numbers or outliers in your sales data can distort your demand model predictions. If continued unchecked over a period of time these errors can have a domino effect on your business and affect your bottom-line.
Data prep work typically involves several iterative, ongoing processes including, but not limited to:
- Data collection: The first step involves collecting the data sets relevant to the business problem you are trying to solve and setting up a connection to a database/API so the extracted data can be loaded into a common repository.
- Data extraction and loading: This involves ensuring the completeness and accuracy of data sets, by removing/fixing missing values, nulls, or outliers to prevent them from skewing analysis results.
- Data cleaning: Depending on the AI use case identified, data labelling may also be required. This includes identifying raw data and adding one or more meaningful and informative labels to provide context so that an AI model can learn from it. This data-centric approach to AI ensures that better results are delivered.
- Data labelling: Depending on the AI use case identified, data labelling may also be required. This includes identifying raw data and adding one or more meaningful and informative labels to provide context so that a ML model can learn from it.
- Data transformation: This may involve normalizing variables in your data so it doesn’t disproportionately influence the AI model, and ensuring that qualitative data (such as product categories or customer segments) may also need to be encoded into a numerical format so that your data is in a unified and usable format that can be fed into the algorithms.
Data preparation is an ongoing process that can involve many more steps depending on the purpose of your analysis. As AI models evolve or when new data becomes available, data professionals will have to revisit their data prep process.
“2024 will see far greater activity around securing the right digital foundation from which to explore and build AI-focused initiatives. Without the right data and the right context, AI is difficult to fully capitalize on.”
Gaurika Wijeratne, Vice President, Data & AI, Fortude
Step 3: Building a centralized repository for all your analytics needs
From keeping up with growing data volumes and integrating cloud and on-premises data, to data quality problems, streaming real-time data and unifying inconsistent data silos, there are many analytics challenges to navigate. Therefore, this step of bringing together data from disparate sources and storing it in a single, centralized place is a crucial one in your analytics journey. While there is no single approach, it is important to note that it must be an ongoing process as organizations will continue to accumulate more data over time.
- A data lakehouse combines the benefits of data lakes and data warehouses to improve processing time and efficiency without compromising on flexibility. This means that it essentially enables the storage of raw data like a data lake, while also facilitating the option for preprocessed, structured data like a warehouse.
- Data lakes which function as centralized storage repositories that store large amounts of raw data that may include structured, semi-structured, and unstructured data. This means that businesses can work with data without being held back by constraints related to schema or structure.
- Data warehouses re-processes and stores data in a structured way which makes it easier for businesses to use and understand data. However, the drawback in this mechanism is that it comes with limitations on how organizations can use this data for analysis, and it may not be best option for certain AI scenarios.
Step 4: Defining your unified analytics layer
Once all the data has been cleansed and processed and stored, it enters the realm of the end consumers of data who harness the value of this data pipeline through interactive exploratory analysis, reports, data visualization, data science and statistical modeling. Thus, the analytics layer helps business leaders derive insights from their raw data and make evidence-based decisions. This also becomes the referential point for Co-pilot and AI models to work off and produce predictive and prescriptive analysis.
Operating between the storage and consumption layers of the data analytics stack, is the business semantics layer which gives context to your complex data and removes the technical complexity of data analysis making it easier for business users to draw out insights. While Al and ML are powerful, they are not without limitations. This is where the semantic layer has a role to play in helping the models perform tasks more effectively by ensuring that the data can be easily used by the model as it is from a cleansed, validated source with meaningful business semantics. This also becomes the referential point for Co-pilot and AI models to work off and produce predictive and prescriptive analysis.
Step 5: Establishing a data governance foundation for the AI era
The release of generative AI models over the past few years has put AI at the center stage in the global policy debates. But what does this mean for companies looking to adopt AI initiatives? Businesses must start off with a unified approach by cataloging and inventorying structured and unstructured data so users can recognize the source, sensitivity, and lifespan of every piece of data that is used. It is also crucial to identify high-risk data combinations (such as the combination of customer identification details and their credit card number) and take necessary actions to lower the risk.
Data privacy regulations, security frameworks and AI ethics guidelines continue to evolve even at this very moment. Business leaders must, therefore, make a concerted effort to continuously assess their data against these developments and put in place the right policies to detect violations, mitigate risks and apply corrective action.
Making AI your digital transformation ally
Your algorithm is only as good as the data it is trained on. To truly benefit from the AI wave, businesses must focus on their entire data lifecycle. This begins with establishing a robust data analytics infrastructure to ensure that the data you are feeding the algorithm is accurate, complete and devoid of anomalies that may affect the outcomes. A culture of continuous data refinement and strong data governance frameworks are not just prerequisites, but they are also the linchpin on which AI success hinges.
Take for example, the case of a leading apparel manufacturer and supply chain leader to global fashion brands that partnered with Fortude to invest in their data analytics foundation. The manufacturer’s analytics adoption strategy has not only brought 60% cost savings on advanced analytics initiatives and considerable savings on infrastructure costs, but has also fast-tracked its decision-making process. Their analytics foundation has also helped eliminate architectural blockers for their near-real time data needs, setting them up for AI success.
Ready to build the data analytics foundation for your AI adoption plans? Speak to our team.
- AI adoption: Go all in or take an iterative approach?
- Step 1: Inventorying your data
- Step 2: Prepping your data for analysis
- Step 3: Building a centralized repository for all your analytics needs
- Step 4: Building your unified analytics layer
- Step 5: Establishing a data governance foundation for the AI era
- Making AI your digital transformation ally
Subscribe to our blog to know all the things we do
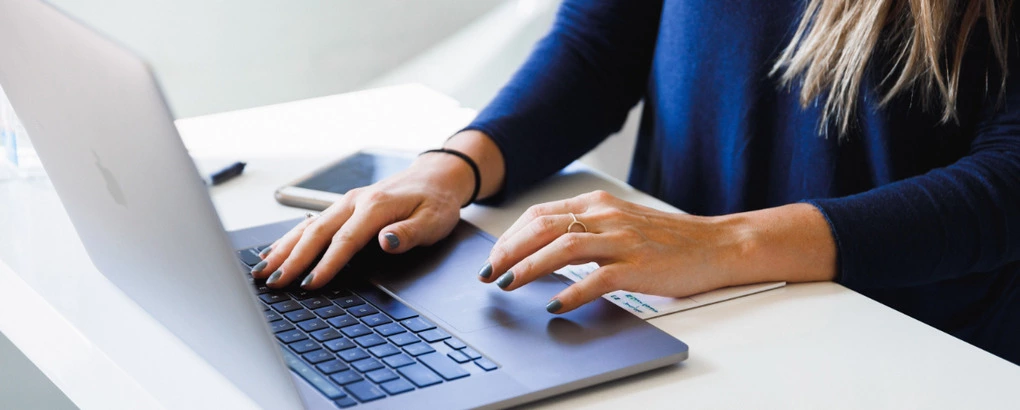